
Machine learning and predictive models for B2B
We help B2B companies to identify decision-making processes with the greatest impact on performance and to improve them through the use of prescribed or predictive data and algorithms
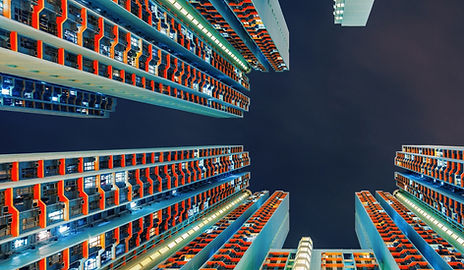
Value for customers
For a long time, companies have collected and managed data without fully exploiting its value. In an increasingly complex market, the ability to combine the experience of management with decision-making processes and prescriptive algorithms driven by data is one of the keys to developing and maintaining a competitive advantage over competitors. We have developed and tested a 4-step approach to improve the quality of critical decision-making processes quickly and with low implementation costs
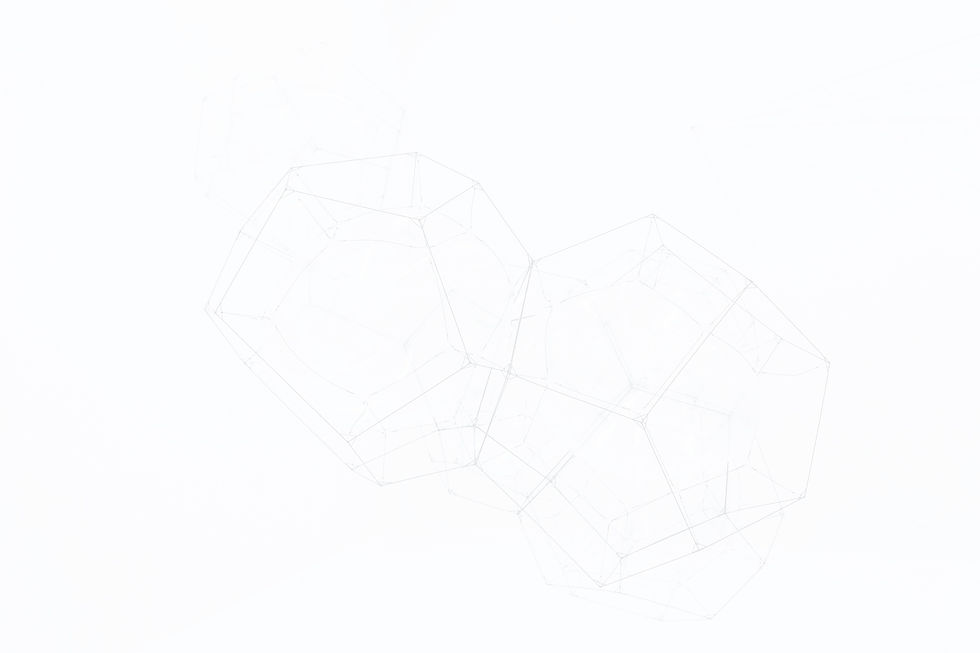
The challenges
The main decisions and challenges companies need to face to improve profitability and successfully compete on the market.
Impact of machine learning models
For which decision-making processes does the implementation of predictive and prescriptive algorithms have the greatest impact and the greatest ROI?

Which technologies allow us to optimize the development costs of machine learning models and reduce dependence on external suppliers
Development costs

Data and feature engineering
How can we get the most value from internal data? In which cases is it appropriate to also use external data?

Internal skills
What skills do we need to equip ourselves to develop the full potential of machine learning while minimizing management costs?

How we help our customers
Critical processes
Diagnostics:
Process
Responsibility
Prescriptive / predictive model
Motivation for action
TO BE process design
Process Diagnostics
Assessment (objectives, process discretion, Data Readiness Level)
Technology selection: self-service software, enterprise software, open source systems
Program set up
Execution support
Data-drive capability development
business problem
business case
Data enrichment and feature engineering
Model test
Pilot deployment
Deployment and training
Development of prescriptive models
Expected results

Decisions and impact on performance
Application of a diagnostic framework for decision-making processes based on 4 evaluation areas

Levers for improvement
Implementation of predictive / prescriptive models with predictive capacity testing and deployment
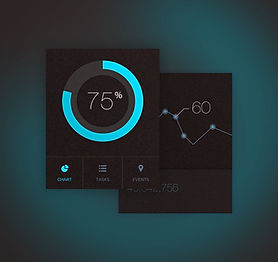
Self-service technologies
Application of self-service machine learning software to reduce costs and dependence on external suppliers
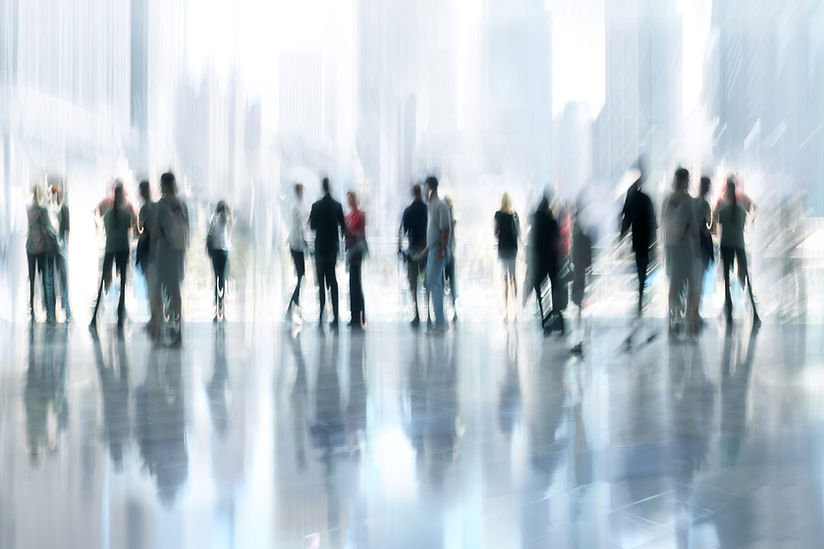
Why Alyant

Approach : field-tested approach based on 4 areas of intervention: 1. Process, 2. ownership. 3. Data and Machine Learning model 4. Motivation for action

Technology : use of Advance analytics self-service software for fast implementation times and low development costs

Autonomy : reduction of dependence on external suppliers for the management and development of new algorithms (thanks to the use of self-service software)